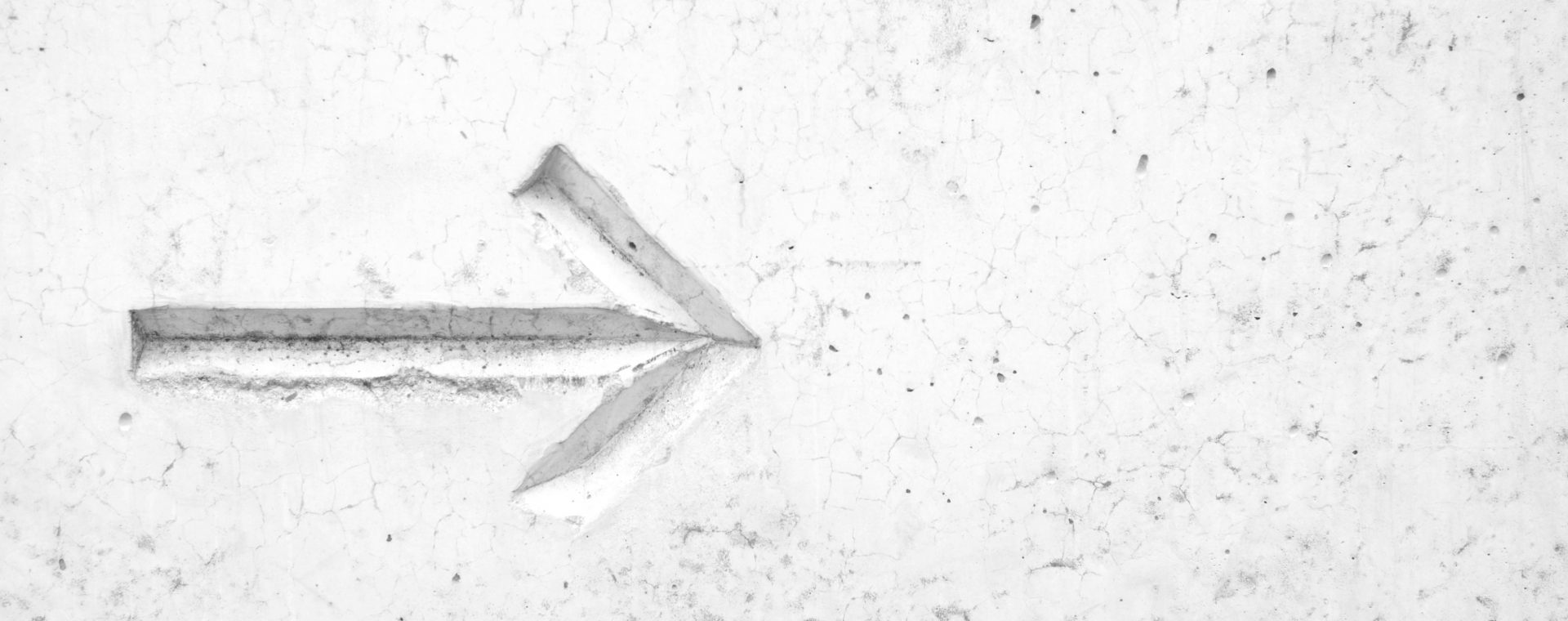
As schools strive to be relevant and globally connected, school reform takes on both local and international contexts. International contexts have become widely associated with comparative results from international tests, such as the Trends in International Mathematics and Science Study (TIMSS) and the Programme for International Student Assessment (PISA), which purport to measure certain aspects of educational quality. New ways of looking at school reform involve a deeper understanding of contexts and non-academic outcomes, including economic, social, and environmental outcomes.
Contexts that determine future outcomes
Fundamental shifts in the economy, the social sphere, and the environment at the global, regional, and national levels are redefining how we work, live, and learn in Singapore. For example, rapid technological advancements globally are reshaping business models where organizations compete on intangible assets such as intellectual property, data and user networks. Another shift is Singapore’s ageing population and low birth rate. This demographic change will lead to a shrink in the growth of the local workforce and place strain on the social fabric of Singapore. Also, Singapore faces the challenge of maintaining peace and harmony in its religiously diverse society. On the environmental front, Singapore needs to balance its urbanization need for resources with sustainable practices to reduce carbon footprint. These contexts—economic, social and environmental—have great implications for the future outcomes that we want our education system to develop in learners.
Future-ready outcomes to meet the contexts
The following table summarizes the trajectories of Singapore’s economic, social and environmental contexts and the skills, knowledge and values required of learners for these trajectories.
Context |
Trajectory |
Skills, Knowledge, Values |
Economic |
|
|
Social |
|
|
Environmental |
|
|
Literature on measuring future-ready outcomes
Measuring the future-readiness of learners is crucial in building a successful future-ready initiative. There are many options to measure future-readiness. The key here is that a single method of measurement is unlikely to provide a comprehensive snapshot of all the aspects of future-readiness of an individual, a school, or a system. Triangulation is important because different measurements study different aspects of the construct to give a fuller picture. A number of scholars have described ways by which 21st century skills, a construct related to future-readiness, might be assessed. Generally, the methods can be classified into the following—self-rated, direct-assessment (multiple-choice, close-ended computer-based items), open-response, teacher judgement and reporting.
Potential of an analytic model to measure future-ready outcomes
Modeling can be used for measurements of non-traditional educational outcomes. Machine learning is a method of data analysis (modeling) that automates analytical model building. It is a branch of artificial intelligence that is based on the idea that systems can learn from data, identify patterns and make decisions with minimal human intervention. Machine learning has made much recent progress due to the development of new learning algorithms, theory, and by the explosion of data and availability of low-cost computing. The explosion of data nowadays demands methods that are capable of handling them adequately and efficiently. Humans are prone to error when manually handling large amounts of data without automation; hence, machine learning has a significant edge over traditional methods of data handling in this respect. Machine learning, therefore, fits well with the dynamic systems theory where system boundaries in an educational organization interact asymmetrically and interconnectedly to impact learner quality outcome measures.
Successful school reform must include outcomes that fulfill all three purposes—economic, social, and environmental—with a keen awareness of the future perspectives of these purposes. Future-ready learners will need to learn deep competencies and cultivate habits of practices that will continue to be valuable long after graduation as the future landscapes of Singapore continue to evolve and change.